Fantasy Football and Sample Size: The Validity of Advanced Analysis
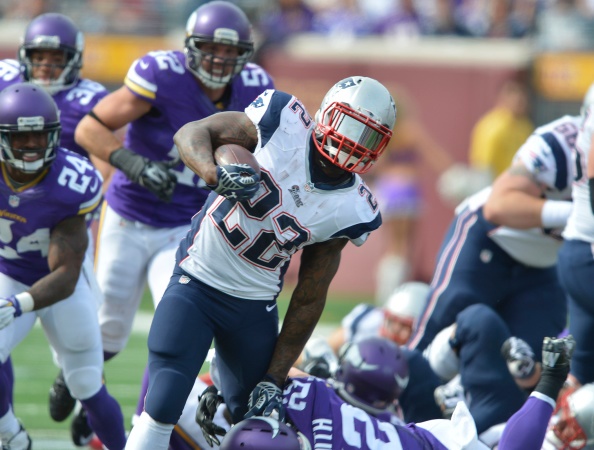
Let’s begin with an apology. The intent was to post my regularly scheduled DFS football strategy piece on Tuesday. The topic was going to be the best way to fill your flex, looking at the safety of a using a running back for cash games versus the risk-reward nature of a wide receiver for GPPs. But as I started crunching some data, looking at the reliability and variance of the two positions, my mind began to wander, questioning the validity of the sample I was using to generate the results.
So then I switched gears and began writing about analyzing football data and the perils of bias not properly fleshed out via a sample of sufficient size. I kept writing and realized I was no longer working under the mantra of strategy but was hedging more into pontificating. And since it just so happens there’s a zone set up here for when my mind starts heading down that road, I opted to apologize to management for missing my scheduled Tuesday DFS posting and instead spend some bandwidth talking in more general terms about football analysis as opposed to providing means to help win your DFS contests. Though, in a sense the message unto itself is advice.
Anyway, so then I stopped writing about my sample size conundrum and deleted out the original introduction and replaced it with the two paragraphs I just wrote plus this sentence. In a moment, I’ll toggle back down and finish the narrative and post it, but before I do I want to apologize to you as well for missing Tuesday’s DFS posting and promise it won’t happen again – or my Friday DFS posting as well. Now excuse me while I wrap this bad boy up.
I find it ironic. Fantasy baseball wishes it were more like fantasy football and fantasy football wishes it were more like fantasy baseball. Baseball yearns for football’s simplicity and ease of play while football strives to incorporate more advanced metrics to aid in analysis. As someone who has a reputation for having a decent handle on using advanced metrics in baseball, you may intuit I embrace the evolution in football. And I do.
The reason for these advancements is simple and not at all meant as a slight. Football analysis is largely trying to discern how the game will go and meshing player performance to match that expectation. In contrast, baseball is mostly focused on how the player will do based on his history and skills. Granted, DFS baseball takes that and adjusts according to matchup, venue etc. but the majority of the expectation is player based.
My sense is football analysis wants to become more player oriented thus the advent of target metrics, yards per attempt, yards after contact, red zone stats etc. I admit, I look at them as a means to help evaluate player performance.
While I’ve seen some research corroborating a cause and effect nature of some of these football metrics, I haven’t seen the depth of study available in baseball. That is, a lot of the conclusions are based more on intuition than a study showing this particular set of data should result in this outcome. I may be all wet on this and now that baseball season is over I plan on looking into it, but my common sense tells me if this sort of thing existed, it would be quoted and referenced much the way it is in baseball.
But that’s not the biggest problem. Inherent in this sort of analysis is a sample devoid of bias. This is where I have the biggest issue. I’m not convinced there’s any way to parse the NFL games such that the data is predictive. How many games are necessary before we can truly say this team is good against defending the run in the red zone or is soft against tight ends? Even if there is a number, what do we do before that? Are last season’s games applicable? Can they be carried over or do coaching and/or personnel changes render last season moot?
Think about the NFL schedule. Not only is it unbalanced, the non-division teams you do play are either home or away. I am quite familiar with the studies that show strong winds are the main issue with weather, but climate further skew this imbalance. There are games played in domes, on turf, on grass, in 80 degree heat and on a frozen tundra.
Now think about how one injury can alter the personality of a team. One team plays a game against a starting quarterback while another faces the back-up, yet both of these games carry the same weight as the data is crunched.
Football, more than any other sport involves game-planning which can alter the distribution of stats. Perhaps a team schemes secondary coverage to blanket the wide receivers, leaving the tight end free for a big game. In a vacuum, that team struggles against tight ends so Jimmy Graham will no doubt go off on them. What happens? The next week the game-plan is to bracket Graham and take him out of the offense, giving the sideline patterns to the receivers. Again, both of these games are thrust into the same data pool.
Finally, one play can change the entire complexion of a football game. An early defensive or return touchdown can alter the flow of a game. A great example of this was the New England Patriots victory over the Minnesota Vikings a couple weeks back. The Pats defense helped build a big lead so on offense, Stevan Ridley was called upon to protect the lead and keep the clock moving while Shane Vereen watched from the sideline. Much of the analysis the following week suggested the Patriots would get out to another big lead with the implication Vereen would be a weak play. Disregard how the game turned out, a close affair. The fault in the reasoning concerning Vereen was while it was quite possible New England would get a big, early lead, it wouldn’t be via a pick-six and other turnovers like it was against the Vikings. No, it would be the offense driving for the touchdowns. And if the offense did score early and often, Vereen would have been involved. Sure, Ridley likely would have closed again, but Vereen would have done some early damage. The point is, even with all the other factors previously discussed, one or two plays can alter the dynamics of a game but this game is also lumped in with the rest.
On one hand, I agree; football needs more advanced statistical analysis. But on the other, I don’t trust that the database inventory is sufficient to render conclusions that are statistically significant.
I don’t know. I listen to analysts that incorporate some advanced numbers into their analysis but I’m not convinced their advice is based on anything more than subjective considerations. I think there’s a lot of writers trying to be the smartest guy in the room, using these stats without any basis. But man, it should sure sounds or reads good.
I realize watching games helps alleviate this problem, but it does not totally eliminate it. There’s too many games and too many nuances to pick up on everything. That’s what stats are supposed to do. Stats are supposed to tell the story in lieu of watching the game. They do the job, not completely, but to a large extent in baseball. I just don’t think the imbalanced schedule lends itself to the same level of analysis in football.
Sigh. I’m starting to make circular arguments so that means it’s time to bring this to a close. In baseball, there are some elegant studies that show when the baseline expectation of each skill stabilizes. That’s what’s needed in football. How many games are needed before we can quantify a defense against the pass or run defense in the red zone? This is a project I want to investigate as it is integral to not only DFS strategy but traditional fantasy football as well. Without knowing that, there’s a lot of subjectivity involved with analysis. And like I alluded to, there’s nothing wrong with that so long as you don’t pretend it isn’t. Using advanced stats means nothing if their foundation isn’t devoid of variance.
Sorry, but I don’t think it is.